From Random Forests to Gradient Boosting: A Journey Through Ensemble Learning
- July 25, 2023
- Posted by: Kulbir Singh
- Category: Artificial Intelligence Data Science
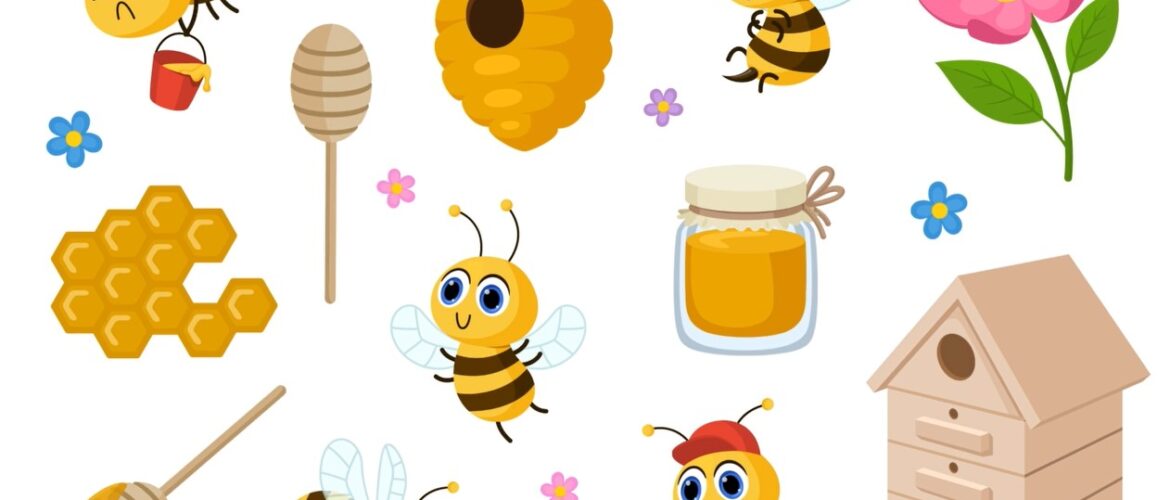
Ensemble Learning
Ensemble learning is a powerful machine learning concept that involves combining several models to produce predictions that are more robust than those of the individual models. The idea is to reduce bias and variance, making the model more flexible and less sensitive to specific data.
For example, imagine you’re trying to predict the weather for tomorrow. Instead of relying on a single forecast model, you use several models (like a decision tree, logistic regression, and a neural network). Each model makes its own prediction, and the final prediction is based on the majority vote of all models. This is the essence of ensemble learning.
Two popular ensemble methods are bagging and boosting:
Bagging: This method involves training several models in parallel, each on a random subset of the data. The final prediction is based on the majority vote of all models. A common example of bagging is the Random Forest algorithm.
Boosting: This method involves training several models sequentially, where each model learns from the mistakes of the previous one. The final prediction is a weighted vote of all models. Examples of boosting algorithms include AdaBoost and Gradient Boosting.
Bagging
Bagging methods work best on high variance, low bias models (i.e., complex models). This is because bagging methods aim to decrease the model’s variance without increasing the bias. Therefore, bagging methods are usually applied to complex models which tend to have high variance.
Bagging is used when the goal is to reduce the variance of a decision tree classifier. Here the objective is to create several subsets of data from the original dataset (with replacement), training each subset with a decision tree model, and then aggregate the output of all the trees to make a final decision. The aggregation function usually takes the form of voting for classification problems and averaging for regression problems. Bagging is a simple way to create different models without worrying about choosing a learning rate.
Algorithms that fall under bagging include:
- Random Forest
Random Forest
Random Forest is an ensemble learning method that operates by constructing multiple decision trees at training time and outputting the class that is the mode of the classes (classification) or mean prediction (regression) of the individual trees.
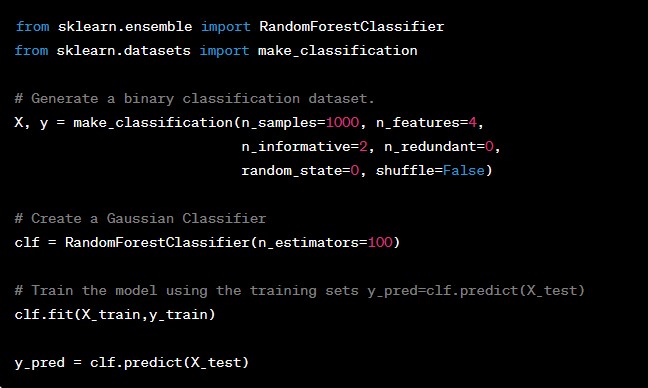
Here’s a step-by-step breakdown of how Random Forest works:
- Step 1: Select n random subsets from the training set.
- Step 2: Train n decision trees. One random subset is used to train one decision tree.
- Step 3: Each individual tree predicts the records/candidates in the test set, independently.
- Step 4: The final prediction is the one that receives the majority of votes from all the decision trees.
Boosting
Boosting methods typically work best on high bias, low variance models (i.e., simple models). Boosting methods aim to decrease the model’s bias without impacting its variance. Therefore, boosting methods are usually applied on simple models which tend to have high bias.
Boosting is used when the goal is to reduce bias and also to reduce variance. Boosting operates in a stage-wise way like bagging, but the key difference is that the trees are not fit on completely resampled versions of the original data. Instead, each tree is fit on a modified version of the original data where the modifications at each step are determined by the performance of the previously grown tree. Boosting tends to give higher accuracy than bagging, but it also tends to overfit the training data as well.
Algorithms that fall under boosting include:
- AdaBoost (Adaptive Boosting)
- Gradient Boosting
AdaBoost (Adaptive Boosting)
AdaBoost, short for Adaptive Boosting, is a machine learning algorithm that is used as a classifier. When you have a large amount of data and you want to find a way to classify the data, you can use AdaBoost. It’s called ‘Adaptive’ because it adapts to the data it is currently training on.
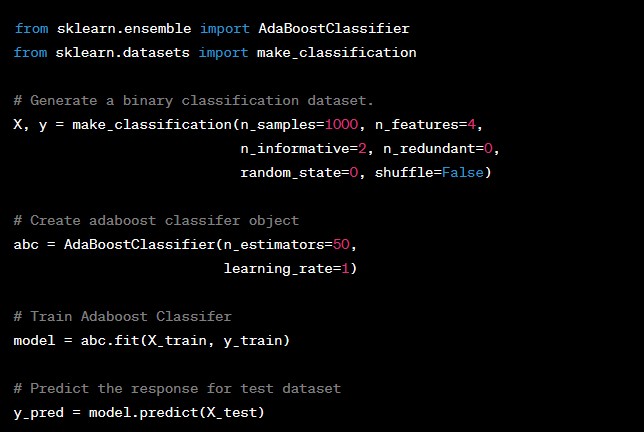
Here’s a step-by-step breakdown of how AdaBoost works:
- Step 1: Initialize the weights of data points. If the training set has 100 data points, then each point’s initial weight should be 1/100 = 0.01.
- Step 2: Train a decision tree.
- Step 3: Calculate the weighted error rate (e) of the decision tree. The weighted error rate (e) is just how many wrong predictions out of total and you treat the wrong predictions differently based on its data point’s weight. The higher the weight, the more the corresponding error will be weighted during the calculation of the (e).
- Step 4: Calculate this decision tree’s weight in the ensemble. The weight of this tree = learning rate * log( (1 — e) / e). The higher weighted error rate of a tree, the less decision power the tree will be given during the later voting. The lower weighted error rate of a tree, the higher decision power the tree will be given during the later voting.
- Step 5: Update weights of wrongly classified points. The weight of each data point = if the model got this data point correct, the weight stays the same. If the model got this data point wrong, the new weight of this point = old weight * np.exp(weight of this tree).
- Step 6: Repeat Step 1 until the number of trees we set to train is reached.
- Step 7: Make the final prediction. The AdaBoost makes a new prediction by adding up the weight (of each tree) and multiply the prediction (of each tree).
Gradient Boosting
Gradient Boosting is another boosting model. Unlike AdaBoost, which tweaks the instance weights at every interaction, this method tries to fit the new predictor to the residual errors made by the previous predictor.
Here’s a step-by-step breakdown of how Gradient Boosting works:
- Step 1: Train a decision tree.
- Step 2: Apply the decision tree just trained to predict.
- Step 3: Calculate the residual of this decision tree, Save residual errors as the new y.
- Step 4: Repeat Step 1 until the number of trees we set to train is reached.
- Step 5: Make the final prediction. The Gradient Boosting makes a new prediction by simply adding up the predictions (of all trees).
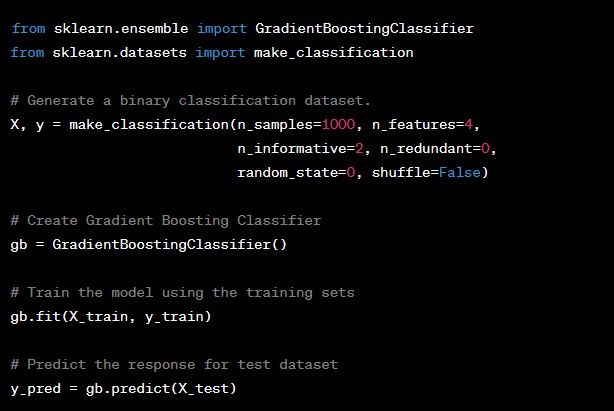
In conclusion, ensemble methods like Random Forest, AdaBoost, and Gradient Boosting are powerful tools for improving the performance of machine learning models. They combine the predictions of multiple models to produce a final prediction that is more robust and accurate.
Autonomous vehicles, also known as self-driving cars, are like smart robots that can drive themselves without a human driver.
Big Data Analytics is like using a magical magnifying glass that helps you see what’s hidden in huge piles of data. Imagine you have a gigantic puzzle made of billions of pieces
TensorFlow is like a magical toolbox that computer wizards, also known as programmers, use to teach computers how to think and learn on their own